Systematic Review of Greenhouse Gas Emissions for Different Fresh Food Categories
Abstract
Informing and engaging citizens to adopt sustainable diets is a primal strategy for reducing global environmental impacts of the agronomical and nutrient sectors. In this respect, the commencement requisite to support citizens and actors of the food sector is to provide them a publicly available, reliable and gear up to use synthesis of environmental pressures associated to food commodities. Here nosotros introduce the SU-EATABLE LIFE database, a multilevel database of carbon (CF) and water (WF) footprint values of nutrient bolt, based on a standardized methodology to extract information and assign optimal footprint values and uncertainties to food items, starting from peer-reviewed articles and gray literature. The database and its innovative methodological framework for doubtfulness handling and data quality assurance provides a solid footing for evaluating the impact of dietary shifts on global environmental policies, including climate mitigation through greenhouse gas emission reductions. The database ensures repeatability and further expansion, providing a reliable science-based tool for managers and researcher in the food sector.
Measurement(s) | carbon footprint of food products and related uncertainties • water footprint of food products and related uncertainties |
Technology Type(s) | digital curation • statistical analysis |
Factor Type(south) | Typologies, sub-tyologies and items of food products |
Sample Characteristic - Surround | agroecosystem • food production arrangement |
Sample Feature - Location | global |
Motorcar-attainable metadata file describing the reported information: https://doi.org/ten.6084/m9.figshare.14115608
Groundwork & Summary
Nutrient is essential for human physical and psychological wellbeing, it is a driver of economy and a key element of cultural identity and human heritage. Food also plays a relevant part in present and future world sustainable development strategies, as the nutrient chain accounts up to 37% of the global greenhouse emissions1, 70% of water withdrawalstwo and strongly contributes to deforestation, mineral depletion, desertification, eutrophication, acidification, biodiversity loss and genetic erosion3,four. Each nutrient product brings its share of generated environmental pressures during nutrient production, processing, distribution and end-lifefive. The degree of such ecology burden varies significantly with food type and with nutrient production characteristics6. Nonetheless, only few indicators of nutrient pressure have standardized procedures, the ii most well-known existence the carbon footprint7 (CF) and the water footprint8 (WF). The CF of a food production represents the total greenhouse gases emitted during all the phases of its production and distribution quantified using a life bike approach. It is a straightforward indicator of the share of global GHG emissions that tin be attributed to food producers or consumers and tin exist easily linked to climate policies. The WF of a nutrient product is the amount of water that is consumed and polluted in all processing stages of its production. Information technology is a consolidated indicator, oftentimes used to compare the relative impacts of unlike food types and dietary habits9.
A global transition towards sustainable food production and consumption is amidst the nearly beneficial global strategies proposed to mitigate human touch on planet resourcesone,3 and it is a central asset of the new Eu strategy "Subcontract to Fork" every bit function of the Eu Green Deal strategy. A clear link has been demonstrated betwixt healthy and sustainable dietary choicesthree, providing a win-win opportunity for all the stakeholders of the food sector, from consumers to food providers. The first step to support societal appointment on sustainable diets is to provide noesis and tools that tin can allow turning ideas into activity. Several studies and belittling reviews of food CF and WF footprints have provided information on dissimilar dietary impacts3,6,10, methodological issues6,eleven,12, policy recommendation6,13. A further step is to offer an open up admission database aimed at unlike kinds of stakeholders, from scientific experts to business companies, citizens and policymakers, to be used for calculations, direction and reporting. To meet this goal, nosotros created a multilevel database of CF and WF of food items, based on a standardized methodology to assign scientifically meaningful values of footprint to food commodities, starting from publications reported in literature. Structure and methodological approach, used to create the database, easily allow for farther expansion or reorganization of specific food items and typologies depending on the specific user'due south need. As such it provides a flexible science-based tool for operators, managers and researcher in the nutrient sector.
The database, adult in the framework of the Eu SU-EATABLE LIFE project, builds on the double pyramid databasefourteen of the Barilla Center for Food and Nutrition. The SU-EATABLE LIFE (SEL) database is organized in different levels of information (Fig. one) suitable for different users and purposes. Level one reports CF and WF information of food commodities extracted from published studies and harmonized to refer to the same arrangement boundaries and functional units. Each data is conceptually associated to a food item name, to a food typology, sub-typology and to a nutrient group (Table 1).
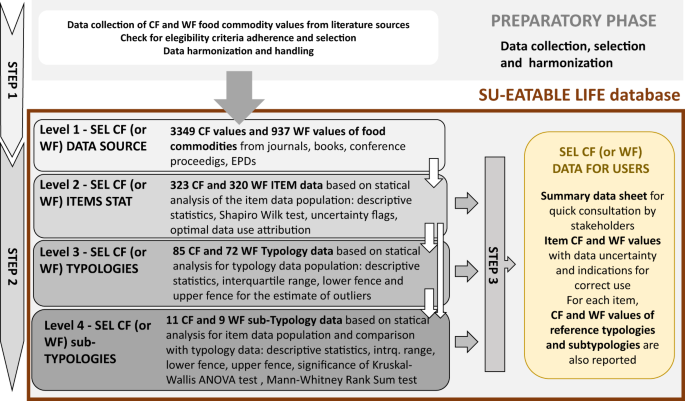
General outline of the different structure steps of SU-EATABLE LIFE (SEL) database. Stride i includes the preparatory stage where studies were collected from literature and public repositories, selected on the footing of eligibility criteria, CF and WF values of food bolt extracted and harmonized, and so reported into the level 1 data of the SEL database. In Footstep 2 the other layers of information are created which stand for different levels of aggregation of data reported in level 1. CF and WF values statistical assay are reported for food items (level 2), typologies (level iii) and sub-typologies (level 4). In Step 3 the circuitous set of data reported in Level, 1, 2 and 3 are summarized into an easy to use dataset suitable for quick consultation by technical and not technical users.
This hierarchical assignment allows shifting hands among different levels of assemblage of food data information. At level 2, information from unmarried studies of level 1 are aggregated to stand for food "item" values and associated doubtfulness, which support the user in determining how robust are the available data for technical and scientific purposes. Level three is a farther level of information aggregation, where detail values from level 2 are aggregated based on their "typology" identifier. Level four is another possibility to represent a food commodity using the value of sub-typologies, which are created when a food typology is quite wide. A distillation of all the information independent in the level 2, three and iv is provided into an easy to use data sheet, SEL CF or WF Information FOR USERS (Fig. i).
Methods
With the aim of obtaining a useful tool for stakeholders to explore, assess and use the information related to CF and WF of nutrient commodities, nosotros implemented a multi-step methodological framework to create an easy to use CF and WF repository of nutrient items, which tin exist expanded or modified for tailored requirements using a science based approach for each footstep of its creation (Fig. one).
The overall methodological procedure is fabricated of 3 steps. Step 1 is related to CF and WF data drove from literature, eligibility check and harmonization, to create the base level of the database (level 1). Stride 2 is about the creation of other 3 informative layers with college level of data aggregation. These might be the information of direct interests for stakeholders of the nutrient systems. A rigorous statistical arroyo is proposed to evaluate the quality of analysed information and criteria for the right utilize of data, based on statistical show, are set and practical to the data. In Pace iii the complex set of statistical evaluations, washed for each informative level, is summarized into an easy to apply dataset reporting values of CF and WF of nutrient items. Cheers to its multilevel approach, the database provides a flexible tool for different purposes and levels of expertise. Each step is based on transparent procedures that permit users to replicate, to implement and to modify each level of the database.
The three steps are described in details in the following paragraphs.
Pace 1 - CF and WF data collection, harmonization and compilation of level i of SEL database
The kickoff step was to review the published data of CF and WF of food commodities. Nosotros revised literature data published till January 2020 including peer-reviewed papers, briefing proceedings, public reports or studies where methods of data collection and handling were described, and Environmental Product Declarations (EPDs).
For the collection of CF data, a significant input came from the systematic review of Clune et al.11, who reviewed 369 published studies, covering the period 2000–2015, proving 168 varieties of fresh nutrient products based on 1718 data entries. An additional source of studies reporting both CF and WF was the Double Pyramid database 2016 built on the previous version 201414 (BCFN2016 https://www.barillacfn.com/en/publications/double-pyramid-2016/), which reports 1202 CF values from 468 sources roofing 240 nutrient items and 309 WF values from 136 information sources covering 152 food items (reference period 1998–2016). Part of CF data of this latter dataset, upward to year 2014, were already revised and included in the Clune et al.11 report. To avert double counting from these two sources, information from both sources were checked for authorship, plus the CF reported data were compared and if in disagreement the original data were checked in the paper. Data reported in the Double Pyramid database 2016 but not present in Clune et al.xi, by and large referring to processed food, were checked for eligibility applying the exclusion criteria reported in Table ii and if considered eligible they were included in the present database.
A new literature search was done to integrate information not covered past the previous reviews using three online bibliographic sources SCOPUS (https://www.scopus.com/home.uri), Google Scholar (https://scholar.google.com/) and the Google search engine (https://www.google.com/), which was concluded in January 2020. To search the bibliographic sources, we used the combinations of two sets of words. The outset set up referred to "impacts" and included the following words: carbon footprint, water footprint, virtual h2o, greenhouse gases, environmental impact, life cycle, LCA, LCI, EPD. The second set referred to "products" and included words similar food, beverages, fish, shellfish, crops, vegetables, fruit, meat, eggs, dairy. EPDs were updated based on information reported on the International EPD's System database (www.environdec.com). Added studies were evaluated for exclusion criteria (Table 2).
The final listing of data from single studies reported in the SEL database was distributed as follow: 3349 CF data, including 1397 information of fresh food commodities already reported in Clune et al.11, 803 CF data originally reported in Double Pyramid 2016 database, which were checked for eligibility and harmonized, and 701 CF data added with this report; 938 WF data, including 288 WF data originally reported in double pyramid 2016 and 650 WF information added with this report.
All the CF and WF values extracted from the nerveless studies were assigned a group, a typology, a sub-typology when this applied, and an item proper name (Table 1) and were recorded on an excel sail including the following additional information: type of bibliographic source, total reference, publication year, system purlieus at distribution, country of production, region of product, relevant notes, presence of the aforementioned value in other information collections (i.e. Clune et al.eleven or Double Pyramid 2016).
After data collection, CF data where further analysed and handled for the harmonization of the system boundary post-obit the approach equally reported in Clune et al.11. The organisation boundary considered in the SEL database is the distribution centre to consumers located in the state of origin. It hence excludes post market phase like for example cooking. The arrangement boundaries at distribution have a wide range of specifications in the published papers. We accepted regional distribution centre (RDC), international distribution heart (IDC), European distribution centre (EDC), country ports of concluding destination, warehouses, wholesalers, city markets, up to retailers. For the specific case of international transport, which includes too the emissions for shipping to regional distribution centres of the hosting country, rather than excluding the studies we take created a dedicated typology "imported", which however includes very few studies. The imported article is indicated in the SEL database by a upper-case letter "I".
If CF values collected from literature referred to the system boundary "farm gate" or "shambles", additional postal service farm gate GHG emissions were added as proposed by Clune et al.11. These boosted emissions also included packaging if not reported in the publication. Nosotros adopted the median value for distribution to RDC (0,09 kg CO2/kg or kg CO2/L) and packaging (0,05 kg CO2/kg or kg CO2/L) used by Clune et al.xi. Data referring to slaughter-house emissions were as well taken from the same publication.
To address the share of WF for packaging and transportation to the market nosotros analysed 256 EPD's. No meaning increase of WF in downstream stages associated to packaging and distribution was found. Thus we included in the assay all system boundaries with the exception of 'cooking', man excretion and waste disposal.
To transform CF values from carcass or live weight to bone free meat, ratios reported in in Clune et al.xi were used, while the ratio carcass weight to bone gratis meat for buffalo meat (1:0.684) was estimated from the studies of Gerber et al.15, Gurunathan et al.sixteen, Li et al.17.
The final version of CF and WF data, after data handling was recorded in a sheet where, in addition to the information mentioned in a higher place for each study, we also reported additional post subcontract gate emissions (send T, slaughtering Southward, packaging P) or meat conversion factors (cf) when applying. This complete dataset represents the level one information sail of the SEL database (Fig. 1).
A modify in 100-year global warming potential (GWP) factors provided by the International Console on Climatic change reports AR3 (2001), AR4 (2007) and AR5 (2013) might have introduced boosted variability in the studies of LCA on which CF information of level 1 are based. The extent of such variability is difficult to quantify equally information technology depends on the relative weight of each GHG on the full CF of the detail. However, the analysis of some item groups (tomato, rice, beef meat, chicken meat), used as sample test, did not bear witness any articulate tendency of CF average reduction or increment over the years (1998–2020), suggesting that differences among production processes and conditions were the dominant source of CF variability.
Step 2 - Creation of derived CF and WF datasets with college aggregation level (two, 3 and 4)
This step provides footprints of food bolt with a higher level of aggregation respective to food items, typologies and sub-typologies (Table 1), which might be of detail interest for different kinds of stakeholders. The particular represents the higher detail of aggregated footprint data of a food commodity and it is ofttimes the virtually desirable information for food impact analysis and dietary assessments. We propose here a methodological framework to evaluate the incertitude associated to data used to stand for food items. The methodological framework will support the users in their pick of the optimal value to stand for the food item on the basis of the available data nowadays in the database. It also would easily allow for expansion and implementation of food item values.
Level 2, SEL CF ITEM & SEL WF Detail datasets
These ii datasets (CF and WF) written report a comprehensive set up of descriptive statistics for the list of nutrient items present in the database. The population of data used to attribute a value and doubt to a nutrient item is made of all the CF or WF values classified with that "item entry name" in the dataset of level 1 of SEL database.
The item data population is described in level ii by the following set of information.
Size: number of studies used for the analysis of item population (northward).
Location and central-tendency measures: in terms of mean, median, first quartile (Q1) and third quartile (Q3), including as well the minimum (Min) and maximum (Max) observed values.
Variability measures: Standard Deviation (SD) Coefficient of Variation (CV) as absolute and relative dispersion indexes, the Interquartile Range (IQR) and the Median Accented Divergence (MAD) as more robust indexes of variability.
Shape measures: Skewness (SK), kurtosis (KU) indexes and Shapiro-Wilk normality test (SW exam).
The median of the detail information population was chosen to assign a value of central tendency which represents the item. The median offers the reward of not beingness influenced past the presence of outliers which misrepresent the value of the hateful, making information technology a less meaningful measure. As such, the median represents the location estimator with the highest breakdown signal (equal to 0.5) and with "the maximum proportion of observations that tin be contaminated (i.east., ready to infinity) without forcing the figurer to effect in a "faux" and non-representative valueeighteen,19. With these backdrop, the median also represents the nearly appropriate measure of cardinal trend to describe both positively and negatively skewed distributions20.
To depict the doubtfulness associated to the position value (median) nosotros used descriptive statistic data relative to dispersion and shape of item information distribution. In particular, we used skewness and kurtosis indexes, which gave usa information on the existence of symmetric or skewed distributions, as well as on their 'peakedness' measured as relative to the weights of the tails21, thus enabling united states of america to evaluate (for each distribution) the importance of extreme values over the entire set up of data and the related level of dispersion (platykurtic versus leptokurtic distributions). We completed the shape analysis by conveying out the Shapiro-Wilk examination22,23 (4 ≤ n ≤ 2000).
To define the uncertainty of the item value nosotros created an assignment method based on a combination of the three quality flags (Fig. ii).
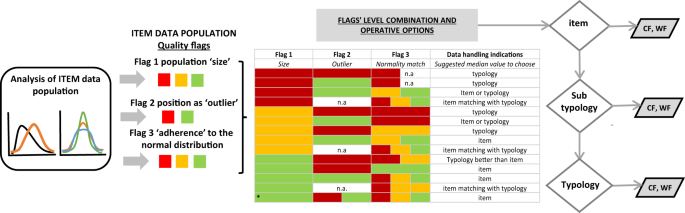
Method for attribution of CF (or WF) value to a food detail based on data quality flags. The scheme shows the procedure applied to evaluate the level of dubiousness associated to CF or WF value of a food detail and how this information is used to decide the best value that should be used to represent the detail. 3 quality flags related to a statistical aspect of the data population are calculated to attribute the level of uncertainty. Each flag has different level of quality, red being the worst, green the best. Flags are and so combined and expert judgement is used to associate a suggestion for data use to each flag combination. If the item median value is characterized by high dubiousness it poorly represents the item and caution is needed to apply this data to represent the nutrient commodity, the users is therefore redirected to a higher level of assemblage such equally the sub-typology or the typology which includes the analysed item.
Flag 1, evaluation of the 'size' (due north) of the "particular data population"
-
Carmine if northward < 4, as three is the minimum number of observations needed for distinguishing the median from the mean and for evaluating the approximation of the empirical distributions to known parametric distributions, in accord with the minimum requirements specified by Royston23.
-
Yellowish if 4 < n ≤ 8, minimum level of observations needed for jointly evaluating kurtosis and skewness of a distribution24.
-
Light-green if northward > 8 number of observations required to place cases in which location (besides as variability and shapes) measures can be properly computed and evaluated.
-
Green with asterisk (*): applies to WF values which are global or regional or national boilerplate estimates and green is assigned independently past "n". In this case the flags 2 and iii do not apply for the evaluation of the WF particular value.
Flag 2, evaluation of outlier position of items characterized past Reddish or Yellow Flag 1
This flag is used to test if items with population size n ≤8, are outliers for their respective typology population. If they are outliers they cannot be alternatively represented by the typology value, as they will exist peculiarly college or lower than the item data population representing the reference typology. An example of this in the SEL database is the CF value of the detail "lobster" which has a yellow flag ane (n = five). The item lobster is an outlier for the typology "shellfish". In this case even if there is a sure level of uncertainty in the "lobster" item value, it is not advisable to substitute this value with the typology value "shellfish".
To aspect flag 2 output, the Tukey's rule25 was used. The outlier identification is based on the quartiles of data distribution, where the start quartile Q1 is the value ≥1/4 of the data, the 2nd quartile Q2 or the median is the value ≥1/ii of the data, and the third quartile Q3 is the value ≥iii/4 of the data. The interquartile range, IQR, is Q3 − Q1. The data used to gauge quartile values are the medians of the items composing the typology population.
-
Cherry if the median value of the analysed item (x), is an outlier, i.e. post-obit the Tukey's rule it is more than 1.5 times the interquartile range from the quartiles, either x < Q1 − one.5 IQR, or x > Q3 + 1.5 IQR.
-
Green flag if the median value of the uncertain item analysed (x), is not an outlier, i.eastward. information technology is within 1.5 times the interquartile range from the quartiles, Q1 - 1.5 IQR < x < Q3 + 1.5 IQR.
-
NA, no flag: the Tukey rule was not practical because the items coincide with the typology, i.e. the typology is only made by this sole item for the time being.
Flag three adherence to the normal distribution
It evaluates the level of dispersion and clustering of the observed information points to the center. To test the adherence of the item information population distribution to the normal distribution, the Shapiro-Wilk test was carried out. The three following colours were assigned:
-
Red: characterizing those items: i) whose size was lower than 4, thus preventing the evaluation of normal distribution approximation, as detailed above; ii) items for which we rejected the null hypothesis of bell-shaped distribution at the 1% level of significance (p-value < 0.01), therefore highlighting substantial asymmetric distribution and/or a heavy-tail distributions characterized by a level of clustering (depression or high) non acceptable to describe the findings with a constructed measure, computed at the same item level.
-
Yellow: for those items whose empirical distribution, even if departing from the Normal distribution, lead u.s.a. to reject the null hypothesis with a greater level of errors (0.01 ≤ p-value < 0.05).
-
Light-green: for those items whose empirical distribution lead us to non refuse the zilch hypothesis, therefore confirming the validity of fundamental tendency measures (at the item level) to be used for summary description.
-
NA no flag: the Shapiro-Wilk test could not exist run due to an insufficient number of data in the population (due north < 3).
The outputs of the three flags were considered together to evaluate the uncertainty related to the median CF or WF value of food items, and based on the level of uncertainty indications for an optimal data utilize were provided (Tabular array 3).
A brief rational of the data handling indication is as follow:
- ane.
item: the item statistics are sufficiently robust. The user tin can utilise the item median value to represent that food commodity.
- 2.
item or typology: although the population used to derive the median of the particular is reduced in size and its distribution does non optimally fit a normal distribution, the median of the detail is non an outlier for the typology population, i.e. the value of the item in non exceptionally high or low compared with other items present in the typology of reference. The user can utilise either the item median value or the typology median value to assign a footprint value to the chosen detail.
- 3.
particular matching with typology: the typology coincides with one single detail, the two objects particular and typology, represent hence the same nutrient article. The user volition discover the same median value in the Item and Typology tables, the option is hence univocal. In this case the level of incertitude can exist estimated from flag one and flag iii because flag two cannot exist calculated (n.a.).
- 4.
typology ameliorate than item: different statistical combinations could atomic number 82 to this option. The doubt associated to the item value is sufficiently high to prefer the typology value to stand for the food commodity although the item value is non to exist discarded.
- 5.
typology (or sub-typology): the high dubiety suggests precaution in using the particular value to represent the food commodity and alternatively a college level of assemblage for this food commodity can be used. When the indication suggests both typology or sub-typology is because there is no statistical difference between the 2 values and the user can cull which one to use.
Level 3, SEL CF Typologies & SEL WF Typologies
In this informative level descriptive statistics is reported for CF and WF data of food typologies. The CF or WF information population of "typologies" is composed by the CF or WF median values of each item that is included in the typology. The value 'n' hence represents the number of items in the typology. The aforementioned statistical parameters reported for the item relative to size, location and central-tendency measures and variability measures are reported for typologies. The flag approach is not used to test typologies considering independently from the uncertainty there is no other meaningful footprint data at higher hierarchical level that can be attributed to a food commodity without losing its specificity. The users can cull to create dissimilar typologies from the ones proposed using CF and WF data provided at level 2 (items).
Level iv, SEL CF sub-Typologies & SEL WF sub-Typologies
Sub-typologies stand for a subgroup of typologies, which have been used when the typology refers to a broad range of nutrient items which could have very different CF and WF values on the footing of some commodity characteristic.
An example is represented by fresh crop products where the yield per hectare strongly influences the CF value (Fig. 3).
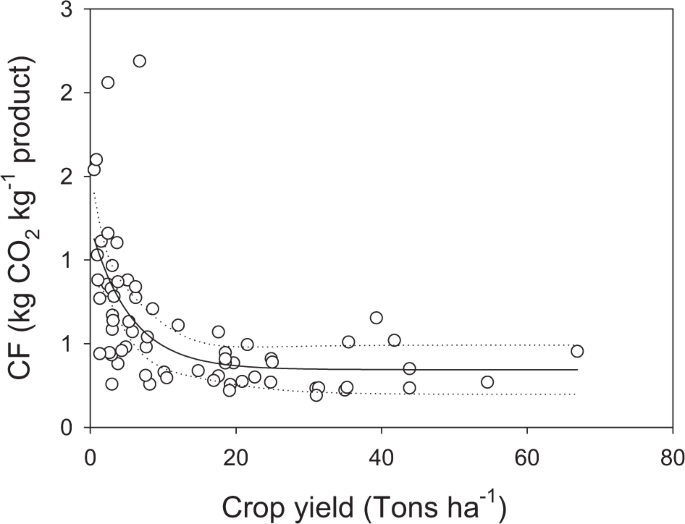
CF value of vegetables vs. their yield. Carbon footprint value of food items included in the typology "vegetables outdoor" is plotted versus their average yield value equally reported in FAOSTAT (data EU-28, yr 2017).
In the SEL database only 3 typologies accept been farther divided into sub-typologies, as they include very different items in terms of their potential LCA outputs. These are vegetable outdoors, fruit outdoor, and shellfish. The population of data used to evaluate the descriptive statistic for each sub-typology is composed by the median of each item that is included in the sub-typology. Additional statistical information in this informative level four are the output of the Kruskal-Wallis ANOVA examination on ranks26 (all pairwise multiple comparing procedures based on Dunn's Method27) to determine if the median values of the sub-typologies within one typology were significantly different from each other, while the Mann-Whitney Rank Sum Examination28 was used to determine if a sub-typology footprint value was significantly dissimilar from its reference typology data population.
Step iii - Creation of summary canvas for easy and quick consultation of CF and WF values of food commodities (SEL CF or WF data for users)
The ii summary sheets (CF and WF information) can exist considered the most interesting and innovative output of the database, as they interpret the complex series of data reported in the 4 informative levels of the database into a listing of footprint values of food based on statistical robust analysis. Footprint values of the food items are represented with additional information about value robustness and, where the uncertainty is high, alternative values with higher levels of aggregation are proposed. The summary sheets are meant to provide a scientifically robust and like shooting fish in a barrel to use tool for experts and not experts who desire to analyse the affect of nutrient bolt and dietary plans. The users are free to accept the good-based suggestions or to make their ain considerations.
Data summary
At present, the SEL database contains 3349 carbon footprint values extrapolated from 841 publications (1998–2019) and 937 h2o footprint values extrapolated from 88 publications (2005–2018). The CF data are summarized into a total of 85 typologies, eleven sub-typologies, 323 items. WF data are summarized into a total of 72 typologies, 9 sub-typologies, 320 items. A detailed breakdown of the CF and WF into the 4 food commodity groups, agricultural processed, animal husbandry, ingather and line-fishing is reported in Table 4.
In terms of geographical distribution, the source information of CF accept a Eurocentric prevalence while the WF data are more evenly distributed among America, Asia and Europe, the relative contribution depending on the commodity group (Table 5).
Potential applications of the database
The SEL database was created based on the necessity to estimate the CF and WF values of nutrient recipes for meals served in canteens during a set of experiments run in the framework of the European union SU-EATABLE LIFE projection aiming at engaging citizens on healthy and sustainable diets to reduce greenhouse gas emissions and h2o use in European union. During the experiments, researchers and canteen managers were faced with the problem of defective a quick tool to summate the food-related environmental impacts based on reliable and scientific discipline-based estimates. The SEL database was created with this purpose and the summary tables take been used for quick conclusion making of sustainable recipes and data management. Following this experience, the SEL database would give a meaning contribution to bridge the gap between the scientific knowledge provided by the scientific community and the actors of the food organization. This historical moment sees a growing attention of the food providers and food consumers to improve the sustainability of nutrient systems. The complexity and challenges of the climate mitigation policies, aiming to reduce greenhouse gas emissions, may discover in dietary shift to more sustainable patterns an additional useful element to achieve the policy targets set out past the Paris agreement.
Many data related to the impact of food bolt are bachelor as public or private repositories or publications, ranging from circuitous scientific studies3,6,11 to online bearding data. The complexity to extrapolate food article data from scientific publications and the necessity to evaluate robustness and scientific value of the data available to users requires from the scientific community and actress effort to provide both meaningful data and a scientific methodology for stakeholders from technical, scientific and policy sectors to easily implement and expand the database with a verified and scientifically sound arroyo. With its different layers of data, the SEL database could hence be a valid tool for to support caterers, chefs, restaurants, nutritionists, municipalities, policy makers, to analyse dissimilar management options related to food and dietary planning.
Database flexibility and directions for database comeback
The SEL database is not an exhaustive collection of CF and WF information for all the possible nutrient items, although the bibliographic search covers a significant part of accessible data sources. The style the database is structured offers the possibility for farther implementation actions:
- one)
Missing items can be included following the methodological framework proposed.
- 2)
Items values and uncertainty can exist implemented by calculation new studies to level 1 and re-assessing uncertainty.
- 3)
Level 1 data could be used for ex novo evaluations.
- 4)
Technical and scientific users might like to make different supposition to further evaluate the doubt of specific items, in this case the articulate and transparent clarification of the steps done to assign uncertainty labels allows to easily take or reject any of the assumptions fabricated in this paper and to implement the overall scheme making new attributions and decisions almost the utilise of the items.
- 5)
The role of geographic data distribution could be evaluated selecting and re-analysing level i information by regional groups, which might exist relevant for indexes like the WF which are driven by climatic factors. The uncertainty analysis might allow to plant if CF and WF information attributed to an item are even so sufficiently statistically robust to extract scientifically sound information. Regionalization of WF values might be relevant when combined with water scarcity studies for specific geographic areas of production.
- six)
The database flexibility allows to introduce new indexes or footprints past calculation a level 1 collection of published information and including the relative levels 2, 3 and 4 calculated using the statistic recommendations provided in this paper. The aforementioned aggregation criteria proposed for the CF and WF might exist used (groups, typologies, sub-typologies, items) for combined analyses of more than indexes.
Data Records
The SEL database is recorded in an Excel workbook called SUEATABLE_LIFE uploaded on Figshare29. It is composed of an introductory sheet to guide the users through the document plus 10 data sheets, five for the CF information analysis and 5 for the WF data analysis, and 2 additional sheets list the typologies used for CF and WF data assay and their description. The content of the 10 data sheets is here described. All the data analyses presented in this paper were done using the software STATA 14.2.
SEL CF (or WF) for USERS is a canvass that summarizes data in an easy to read class for expert and not expert users. It reports for each food item:
-
the nutrient group, the typology and the sub-typology corresponding to each detail (Table 1)
-
the item CF (or WF) value corresponding to the median CF (or WF) value reported for the same item in the SEL CF (or WF) Detail STAT canvass.
-
the level of uncertainty associated to the value of the item based on the three quality flags reported in the canvass SEL CF (or WF) ITEM STAT. The uncertainty associated to the ITEM footprint value is generically summarized as loftier (H) or low (L).
-
The proffer for the optimal employ of the data, namely if the items data is highly reliable or the dubiety suggests to use a higher aggregation value.
-
The alternative value of CF (or WF) that can be used for that specific item at higher level of assemblage (median of typology or sub-typology)
CF (or WF) DATA SOURCES: reports CF (or WF) values of nutrient commodities nerveless from studies reported in the literature, screened using selection criteria and harmonized to report the aforementioned functional unit and organisation boundary. Information provided in this sheet for each input line are: name of group, typology, sub-typology, item (Tabular array 1), CF or WF value after extraction from the publication and harmonization, blazon of source (journal, volume, conference, EPD), full reference, publication year, system boundary at distribution, country of production, region of production, relevant notes, presence of the same value in other data collections, additional post subcontract gate emissions, transport (T), slaughtering (S), packaging (P) or conversion factor applied (cf).
SEL CF (WF) ITEMS STAT: reports for each food particular the following statistical data: number of item values considered for the analysis (n), mean, median, standard deviation (SD), minimum (Min) and maximum (Max) observed values, Median Absolute Deviation (MAD), first quartile (Q1) and third quartile (Q3), coefficient of variation (CV), skewness (SK), kurtosis (KU) and P value of the Shapiro-Wilk normality test. Additionally, information technology reports the colour assigned to each quality flag (Flag one "size", Flag 2 "outlier", Flag 3 "adherence to normality") and the suggested median value to exist used on the basis of the scheme reported in Table 3. The statistical assay for each item reported in this sheet is based on unmarried data of CF (or WF) classified with that specific item entry name in the canvass SEL CF (or WF) DATA SOURCES.
SEL CF (or WF) TYPOLOGIES: reports for each food typology the following statistical data: number of item values (median) considered for the analysis (northward), mean, median, standard deviation (SD), minimum (Min) and maximum (Max) observed values, Median Absolute Divergence (MAD), offset quartile (Q1) and third quartile (Q3), coefficient of variation (CV), Interquartile Range (IQR), lower fence (LF) and upper fence (UF) for the guess of outliers related to the specific typology. The statistical analysis for each typology reported in this sheet is based on median value of CF (or WF) of items classified nether that specific typology and reported in the sheet SEL CF (or WF) Particular STAT.
SEL CF (or WF) sub-TYPOLOGIES: reports for each nutrient sub-typology the post-obit statistical information: number of detail values (median) considered for the analysis (n), mean, median, standard difference (SD), minimum (Min) and maximum (Max) observed values, Median Absolute Deviation (MAD), kickoff quartile (Q1) and 3rd quartile (Q3), coefficient of variation (CV), Interquartile Range (IQR), lower fence (LF) and upper fence (UF), capital letters indicating significant difference among sub-typology medians within the same typology group based on the output of Kruskal-Wallis ANOVA examination on ranks and pairwise multiple comparison procedures based on Dunn's Method, pregnant differences between the sub-typology reported in the raw and the typology including the analysed sub-typology based on the Mann-Whitney Rank Sum test is besides reported.
Technical Validation
Given the high number of inputs and interconnected steps, each step was undertaken by at least two researchers. The literature review on LCA and WF studies followed the recommendations for collecting and synthesising relevant show reported in PRISMA29 (Preferred Reporting Items for Systematic Reviews and Meta-Analyses) and recommendations for CF information treatment reported in Clune et al.11.
To classify typologies and sub-typologies that might be biologically and agronomical sound we had the specific support of biologists and agronomists.
The clarity of the "summary for users' page" was tested with stakeholders of the food communication sector.
Apropos the choice of quality flags to aspect uncertainty to item data populations different options were evaluated and a group of scientific experts with unlike scientific background (statisticians, agronomists, biologist, ecologists) was involved for a final agreed pick.
To further support the correct choice of the attribution of doubtfulness to each item based on the three flags, numerical data were supported by visual data of the distributions. Frequency distribution plots represented past adaptive kernel (Gaussian) density estimates and box and whisker plots were evaluated for each information population which did not provide immediate positive or negative evidence of reliability based on descriptive statistics and tests results.
Two examples of such visualization and the consistent choices are reported in Fig. 4. Panels a and b (Fig. 4) show the empirical distribution of data population of CF values for the Murphy item, characterized by three dark-green flags. Both kernel density and box-plot confirm the low level of dispersion of the empirical data range, the loftier level of concentration of the values around the centre of the distribution and the low density on both tails. The shape of the density part and the distance in the box-plot between Q1-Q3 and the median ostend the empirical adherence of this distribution to the normal distribution, every bit emerged by the SW test
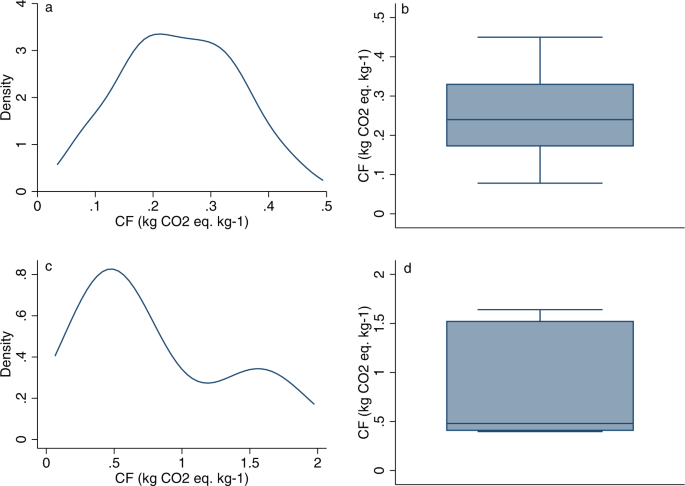
Kernel (Gaussian, bandwidth 0.0433) density gauge (a) and box-plot (b) of spud CF data. Kernel (Gaussian, bandwidth 0.3325) density estimate (c) and box-plot (d) of maize CF data. Empirical distribution for CF data of potato and maize as reported in studies listed in Level 1 of the database (information analysis done with STATA 14.2).
Conversely, panels c and d (Fig. 4) shows the empirical distribution of the CF data population for the item MAIZE characterized by the following flags: "yellowish" for size, "green" for outliers and "ruby-red" for adherence to the normal distribution, which indicated a medium level of incertitude. The number of data in the population (n = 7) is sufficient to distinguish the hateful value from the median value just is not optimal to compute and evaluate variability and shape of the distribution (Flag 3). The graphic visualization of the data in this case supports the attribution of the colour to the Flag3. The stiff asymmetry of the data distribution and the loftier level of overall and cardinal variability, highlighted by the range and the InterQuartile Range (IQR) of the box plot, ostend the median as the most suitable synthetic indicator of the data population and confirm the choice of the crimson flag resulting from the results of the Shapiro Wilk test.
Usage Notes
Researchers and expert users could utilize any level of the database to extract information and could implement the database with additional data post-obit the indications provided in this paper. Users that are non experts of data analysis can direct use the summary sheets 'SEL CF (or WF) data for users'. The sail provides CF or WF values for a listing of items. The users also immediately find in the same raw the CF (or WF) value of typologies and sub-typologies respective to each item. Each item brings a quality indication which refers to the reliability of the data they have in the table. When the user finds the indication that an ITEM value has high dubiety it means that speculations well-nigh this item value demand caution and should be supported by boosted data. For these items the utilise of the typology value might be more than advisable. This could be the instance for case of items represented by very few studies (one or ii) or items represented past few studies (3 or 4) with output values very different from each other. If the users are looking for an detail not included in the item list they tin can choose the median value of a typology or sub-typology of nutrient article which they consider the closest to their missing object.
Users interested in using the methodological framework proposed or data nowadays in the database are asked to cite this manuscript as well as the database and their relative DOI.
Code availability
No custom code has been used for generation or processing of the data or generation of figures.
References
-
IPCC, 2019: Climate change and Country: an IPCC special report on climate change, desertification, land degradation, sustainable land management, nutrient security, and greenhouse gas fluxes in terrestrial ecosystems (eds Shukla, P. R. et al.). https://www.ipcc.ch/srccl/download (2019).
-
WWAP (United Nations World Water Assessment Programme). The United Nations World Water Development Report 2014: Water and Free energy. Paris, UNESCO (2014).
-
Willett, Due west. et al. Food in the Anthropocene: the EAT-Lancet Commission on salubrious diets from sustainable food systems. Lancet (London, England) 393, 447–492 (2019).
-
FAO. The State of the World's Biodiversity for Food and Agronomics (eds Bélanger, J. & Pilling, D.) FAO Commission on Genetic Resources for Food and Agronomics Assessments. Rome. pp 572 (2019).
-
ISO 14044:2006 Ecology management — Life cycle assessment — Requirements and guidelines (2006).
-
Poore, J. & Nemecek, T. Reducing food'southward environmental impacts through producers and consumers. Science 360, 987–992 (2018).
-
ISO 14067:2018(en) Greenhouse gases — Carbon footprint of products — Requirements and guidelines for quantification (2018).
-
ISO 14046:2014 Environmental management — Water footprint — Principles, requirements and guidelines (2014).
-
Harris, F. et al. The H2o Footprint of Diets: A Global Systematic Review and Meta-analysis. Adv. Nutr. eleven, 375–386 (2020).
-
Rosi, A. et al. Environmental impact of omnivorous, ovo-lacto-vegetarian, and vegan diet. Sci. Rep. 7, 6105 (2017).
-
Clune, S., Crossin, E. & Verghese, G. Systematic review of greenhouse gas emissions for different fresh food categories. J. Clean. Prod. 140, 766–783 (2017).
-
Hoekstra, A. Y. & Mekonnen, M. Chiliad. The h2o footprint of humanity. PNAS 109, 3232–3237 (2012).
-
Hoekstra, A. Y. & Mekonnen, Chiliad. Grand. From water footprint assessment to policy. PNAS 109, E1425 (2012).
-
Ruini, 50. F. et al. Working toward salubrious and sustainable diets: the "Double Pyramid Model" developed past the Barilla Center for Food and Diet to heighten sensation about the ecology and nutritional impact of foods. Front. Nutr. two, nine (2015).
-
Gerber, P.J. et al. Tackling climate change through livestock – A global assessment of emissions and mitigation opportunities. Food and Agriculture Arrangement of the Un (FAO), Rome http://www.fao.org/3/a-i3437e.pdf (2013).
-
Gurunathan, Yard., Biswas, S. & Rajkumar, R. Southward. Buffalo as a potential food animal. Int. J. Livest. Prod. 1, i–5 (2009).
-
Li, Q. et al. Effects of age on slaughter performance and meat quality of Binlangjang male person buffalo. Saudi J. Biol. Sci. 25, 248–252 (2018).
-
Donoho, D. L. & Huber, P. J. The notion of breakup betoken. In A Festschrift for Erich Lehmann (eds Bickel, P. J., Doksum, M. & Hodges, J. L. Jr), Wadsworth, Belmont, CA, 157–184 (1983).
-
Leys, C., Ley, C., Klein, O., Bernard, P. & Licata, Fifty. Detecting outliers: Do non use standard deviation around the mean, use absolute deviation around the median. J. Exp. Soc. Psychol. 49, 764–766 (2013).
-
Privitera, G. J. Statistics for the Behavioral Sciences. Sage Publications Inc. (2012).
-
Jones, K. C., Rosco, J. F. & Pewsey, A. Skewness-invariant measures of kurtosis. Am. Stat. 65, 89–95 (2011).
-
Shapiro, S. S. & Wilk, Thou. B. An analysis of variance test for normality (complete samples). Biometrika 52, 591–611 (1965).
-
Royston, P. Approximating the Shapiro–Wilk W-test for non-normality. Stat. Comput. two, 117–11 (1992).
-
D'Agostino, R. B., Belanger, A. J. & D'Agostino, R. B. Jr. A proposition for using powerful and informative tests of normality. Am. Stat. 44, 316–321 (1990).
-
Hoaglin, D. C., Iglewicz, B. & Tukey, J. West. Functioning of some resistant rules for outlier labeling. J. Am. Stat. Assoc. 81, 991–999 (1986).
-
Kruskal, W. H. & Wallis, W. A. Utilize of ranks in one-benchmark variance assay. J. Am. Stat. Assoc. 47, 583–621 (1952).
-
Dunn, O. J. Multiple Comparisons Among Ways. J. Am. Stat. Assoc. 56, 52 (1961).
-
Mann, H. B. & Whitney, D. R. On a test of whether one of ii random variables is stochastically larger than the other. The annals of mathematical statistics 18, 50–60 (1947).
-
Petersson, T. et al. SUEATABLE_LIFE: a comprehensive database of carbon and h2o footprints of food bolt. figshare https://doi.org/10.6084/m9.figshare.13271111 (2021).
-
Moher, D., Liberati, A., Tetzlaff, J. & Altman, D. G. Preferred reporting items for systematic reviews and meta-analyses: the PRISMA statement. J. Clin. Epidemiol. 62, 1006–1012 (2009).
-
Hoekstra, A.Y. The Water Footprint: The Relation Between Human being Consumption and Water Use (eds Antonelli, M. & Greco, F.) The Water Nosotros Eat. Springer Water. Springer, Cham pp. 35–48 (2015).
Acknowledgements
This work was supported by the LIFE program, project SU-EATABLE LIFE Reducing carbon emissions in the EU through sustainable diets grant northward° LIFE16 GIC/IT/000038. The authors admit the back up of Anna Ruggerini, Luca Ruini and Roberto Ciati who developed the Double Pyramid project.
Author information
Affiliations
Contributions
C.Due south. conceived and designed the study strategy; C.S., P.T., S.L., designed the database; S.L. designed and performed the statistical analysis strategy with the support of C.S and P.T.; M.A. revised the food categories and provided support for agronomic data analysis; all the authors supported the literature searches and data collation; P.T. analysed the literature data for eligibility and carried out data harmonization followed by a second revision by C.Due south.; C.Due south., P.T., S.L. carried out data calculations and information summary; C.Southward. took the lead in writing the paper with inputs and reviews from all contributing authors. All authors read, edited and approved the final manuscript.
Corresponding author
Ethics declarations
Competing interests
The authors declare no competing interests.
Additional information
Publisher's annotation Springer Nature remains neutral with regard to jurisdictional claims in published maps and institutional affiliations.
Rights and permissions
Open Admission This article is licensed under a Creative Commons Attribution four.0 International License, which permits use, sharing, adaptation, distribution and reproduction in any medium or format, as long as you requite appropriate credit to the original writer(southward) and the source, provide a link to the Creative Commons license, and point if changes were made. The images or other third party textile in this article are included in the article's Artistic Commons license, unless indicated otherwise in a credit line to the material. If material is not included in the article'south Creative Commons license and your intended use is not permitted by statutory regulation or exceeds the permitted apply, you volition demand to obtain permission directly from the copyright holder. To view a re-create of this license, visit http://creativecommons.org/licenses/past/iv.0/.
The Artistic Commons Public Domain Dedication waiver http://creativecommons.org/publicdomain/zero/1.0/ applies to the metadata files associated with this article.
Reprints and Permissions
Almost this article
Cite this article
Petersson, T., Secondi, L., Magnani, A. et al. A multilevel carbon and h2o footprint dataset of food commodities. Sci Information 8, 127 (2021). https://doi.org/ten.1038/s41597-021-00909-8
-
Received:
-
Accepted:
-
Published:
-
DOI : https://doi.org/ten.1038/s41597-021-00909-8
farringtoncalsomed.blogspot.com
Source: https://www.nature.com/articles/s41597-021-00909-8
Post a Comment for "Systematic Review of Greenhouse Gas Emissions for Different Fresh Food Categories"